Neuro-symbolic ai
Building the Future of Scientific Discovery
Our mission to create the world's first AI Research Factory, combining neuro-symbolic AI with advanced research automation to accelerate breakthrough discoveries.
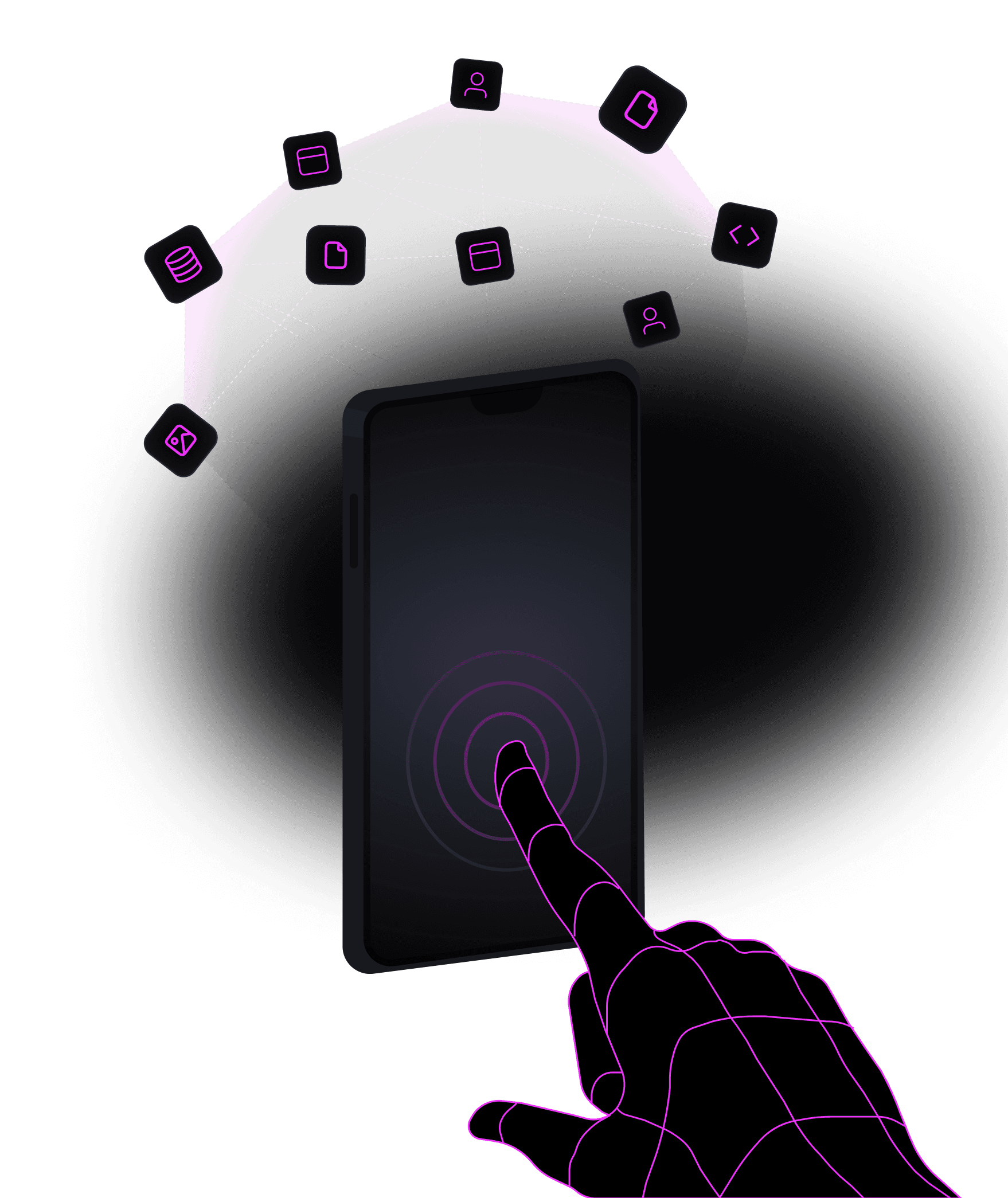
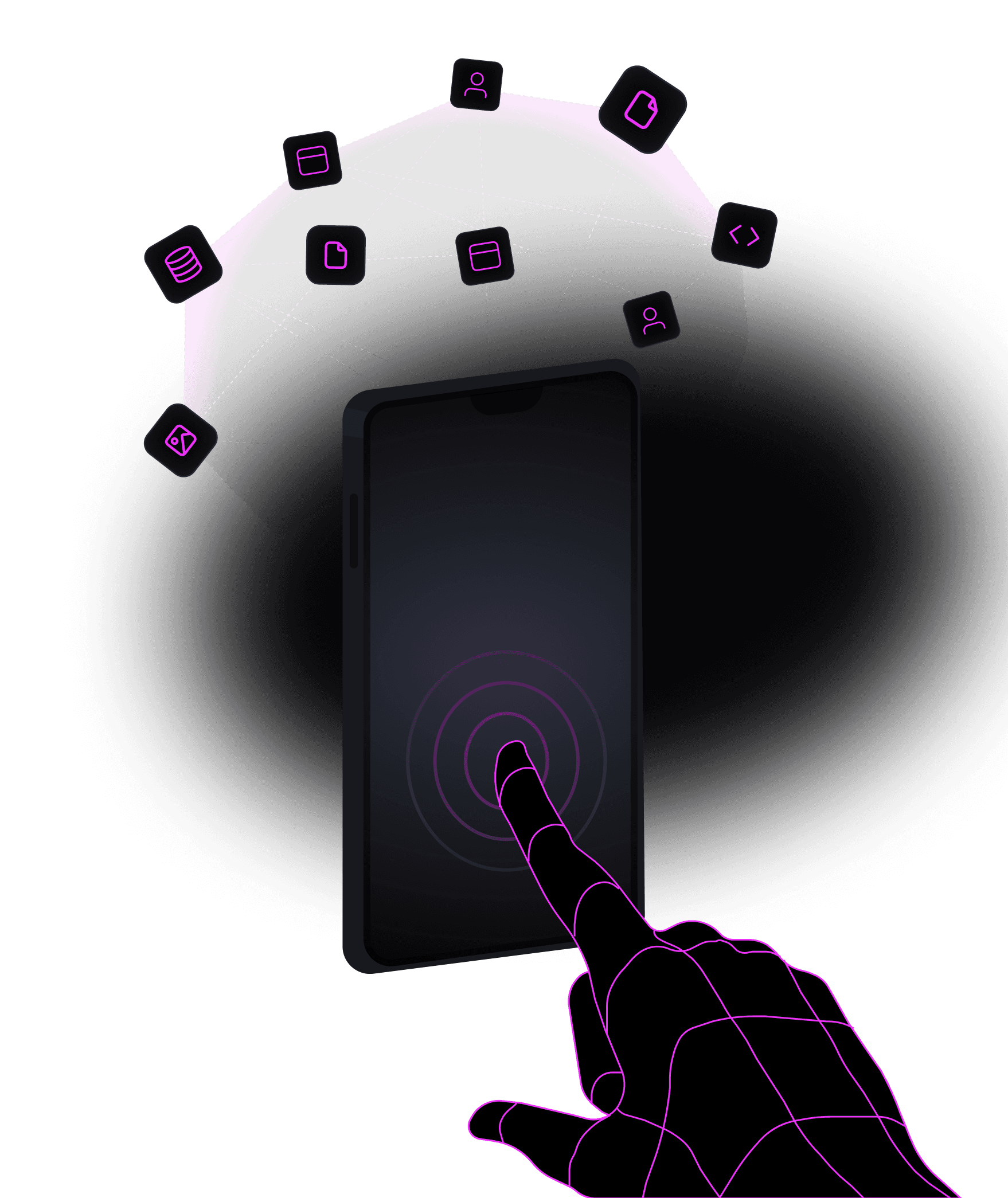
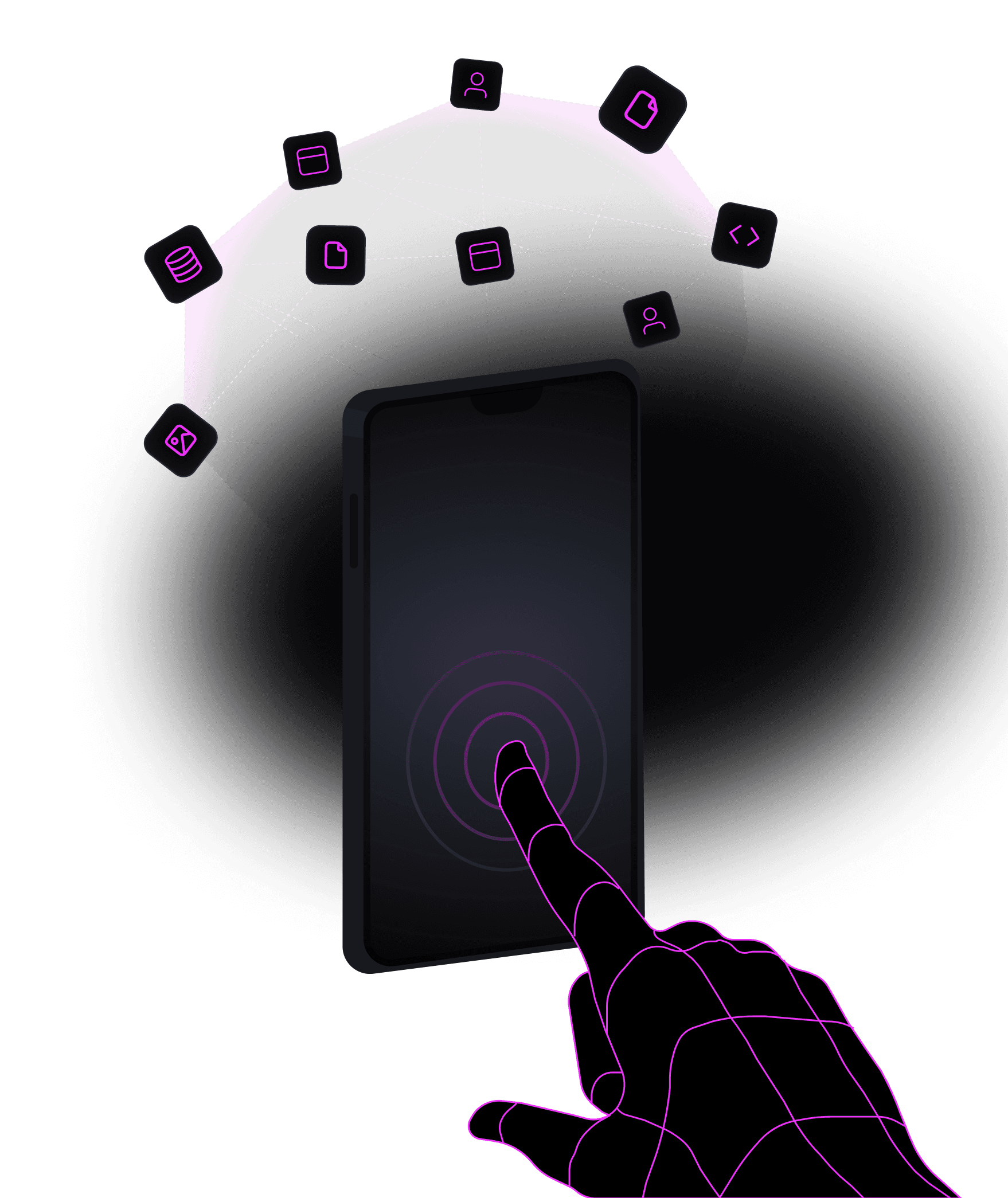
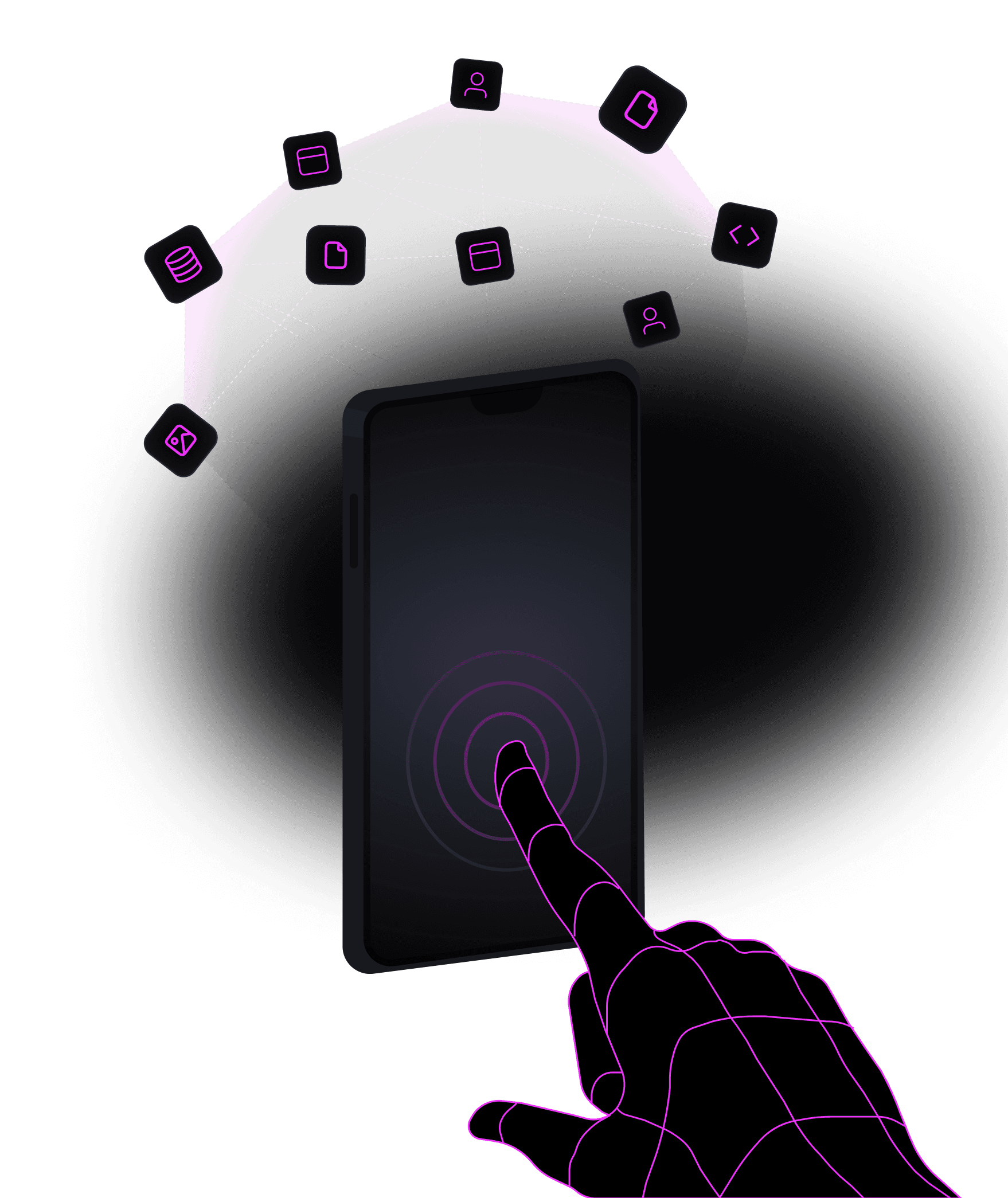
Our vision
Designing Domain Invariant Problem Solvers
The AI Research Factory integrates symbolic reasoning with neural capabilities, enabling multi-step problem decomposition and autonomous research workflows powered by domain-invariant problem solvers.
Our framework orchestrates specialized research agents through traceable computational graphs, where each node operates as a contained, verifiable unit. This architecture enables complex workflows to emerge from simple, reliable components while ensuring the transparency and reproducibility essential to scientific discovery.
Areas of Research
Research Focus
Integrating symbolic knowledge with machine learning capabilities to advance the foundations of artificial intelligence.
01.
Reinforcement Learning
We are developing systems that learn from interactions with their environments, continuously improving their decision-making abilities. This research enables the creation of autonomous agents that adapt dynamically to complex scenarios.
01.
Reinforcement Learning
We are developing systems that learn from interactions with their environments, continuously improving their decision-making abilities. This research enables the creation of autonomous agents that adapt dynamically to complex scenarios.
01.
Reinforcement Learning
We are developing systems that learn from interactions with their environments, continuously improving their decision-making abilities. This research enables the creation of autonomous agents that adapt dynamically to complex scenarios.
01.
Reinforcement Learning
We are developing systems that learn from interactions with their environments, continuously improving their decision-making abilities. This research enables the creation of autonomous agents that adapt dynamically to complex scenarios.
02.
Large Language Models
Our work in large language models enhances AI’s ability to process and understand natural language, pushing boundaries in automated communication, content creation, and decision-making systems.
02.
Large Language Models
Our work in large language models enhances AI’s ability to process and understand natural language, pushing boundaries in automated communication, content creation, and decision-making systems.
02.
Large Language Models
Our work in large language models enhances AI’s ability to process and understand natural language, pushing boundaries in automated communication, content creation, and decision-making systems.
02.
Large Language Models
Our work in large language models enhances AI’s ability to process and understand natural language, pushing boundaries in automated communication, content creation, and decision-making systems.
03.
Neuro-Symbolic AI
By combining neural networks with symbolic reasoning, we’re building AI systems that can reason logically while learning from data, bridging the gap between human-like reasoning and machine learning.
03.
Neuro-Symbolic AI
By combining neural networks with symbolic reasoning, we’re building AI systems that can reason logically while learning from data, bridging the gap between human-like reasoning and machine learning.
03.
Neuro-Symbolic AI
By combining neural networks with symbolic reasoning, we’re building AI systems that can reason logically while learning from data, bridging the gap between human-like reasoning and machine learning.
03.
Neuro-Symbolic AI
By combining neural networks with symbolic reasoning, we’re building AI systems that can reason logically while learning from data, bridging the gap between human-like reasoning and machine learning.
Timeline
Shaping the future of AI
Software 1.0
Refers to classical programming, where people write code manually, defining algorithms, rules, and logic that software must follow. It’s the foundation of how most software has been built traditionally.


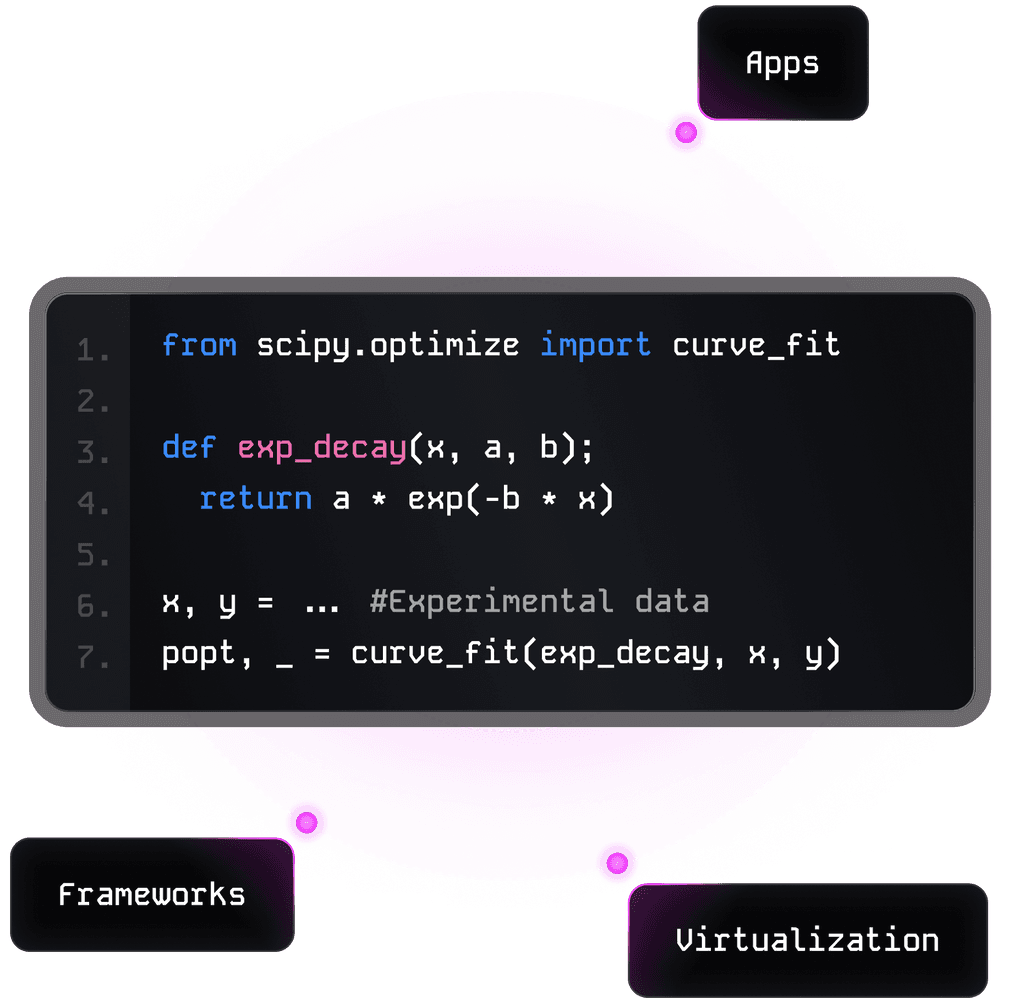
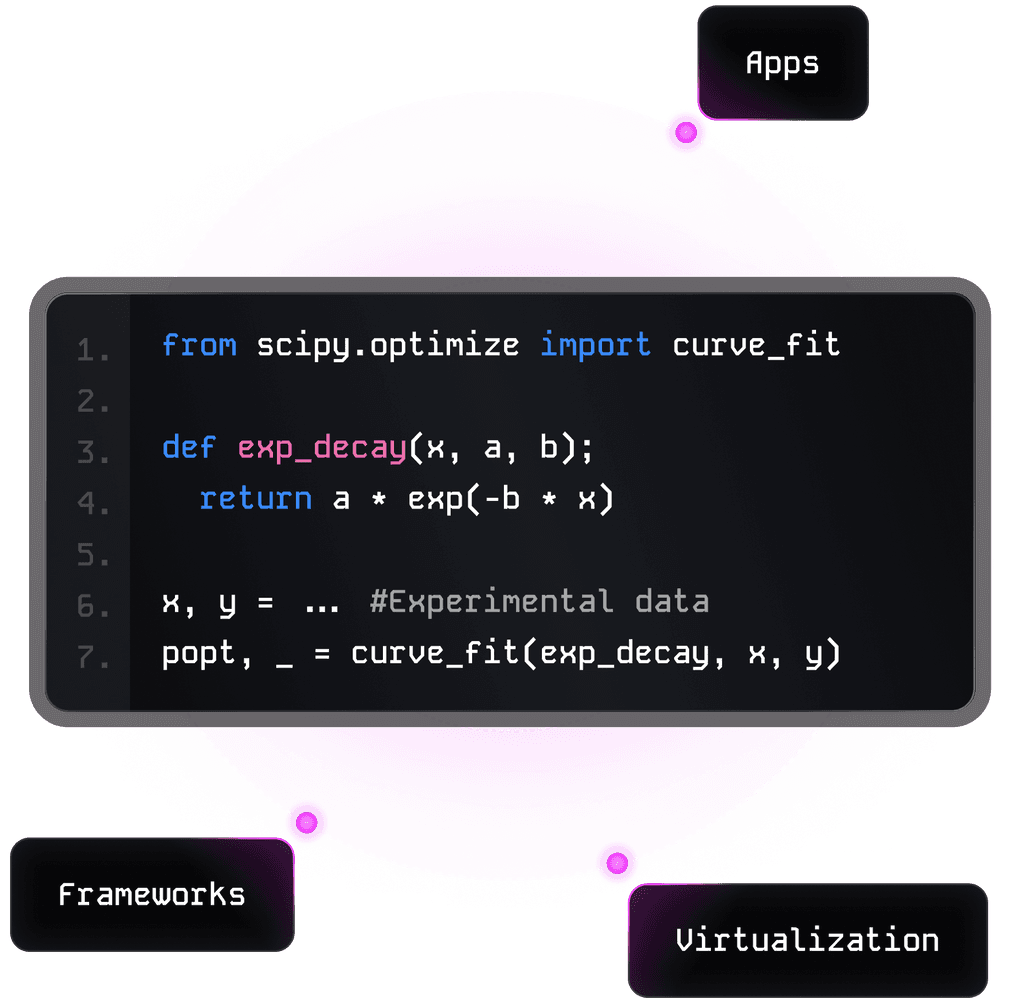
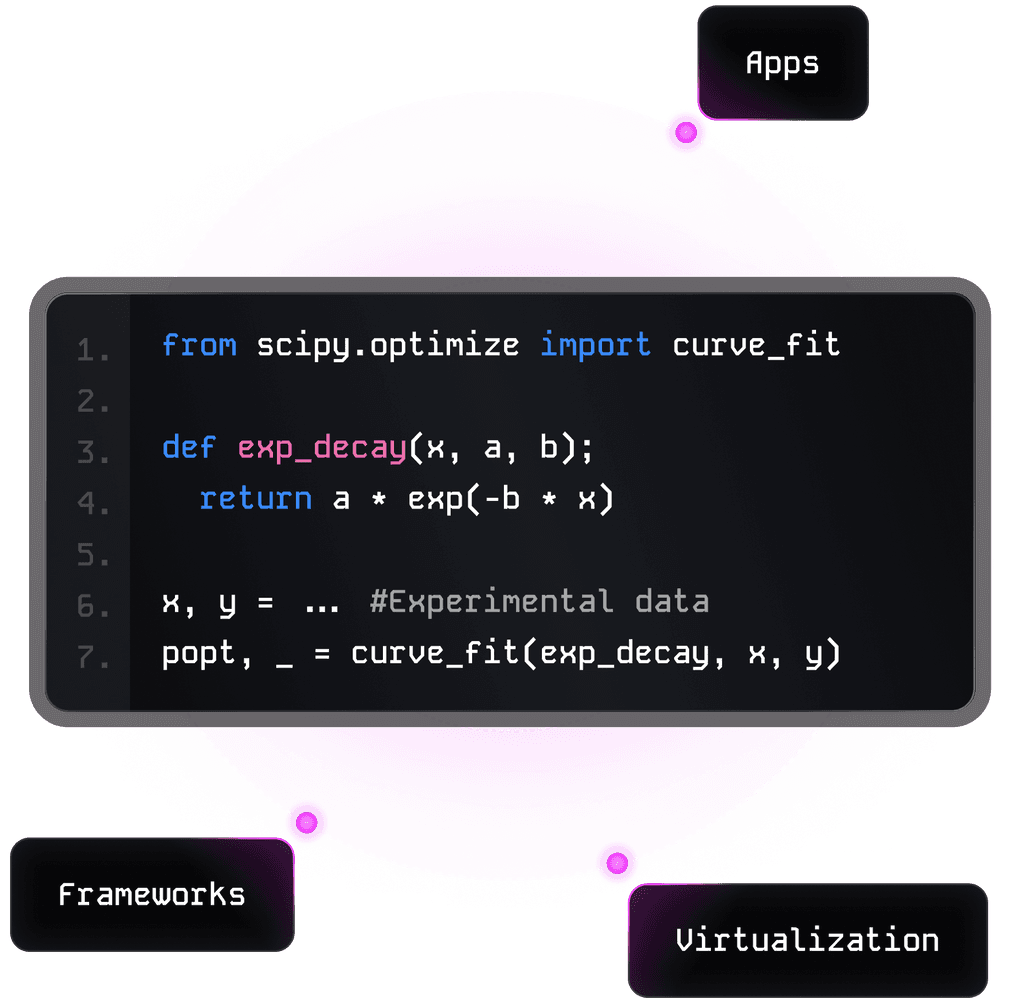
Software 2.0
Represents differentiable programming, where neural networks and machine learning algorithms learn from data. Instead of explicit instructions, these systems optimize themselves by learning patterns, allowing for smarter and more adaptable software.





Software 3.0
The next leap in AI, blending neuro-symbolic programming with reasoning capabilities. This stage builds self-improving algorithms that not only learn from data but also reason and make decisions autonomously.
We aim to standardize how algorithms interact with the world, advancing the field toward fully autonomous AI solutions capable of understanding and improving themselves over time.






Publications
Read the science
behind our technology
Read the science
behind our technology
Last revised: 21 Aug 2024 / Arxiv.com
SymbolicAI: A framework for logic-based approaches combining generative models and solvers
We introduce SymbolicAI, a versatile and modular framework employing a logic-based approach to concept learning and flow management in generative processes.
Last revised: 2 May 2023 / Arxiv.com
Addressing Parameter Choice Issues in Unsupervised Domain Adaptation by Aggregation
We study the problem of choosing algorithm hyper-parameters in unsupervised domain adaptation, i.e., with labeled data in a source domain and unlabeled data in a target domain, drawn from a different input distribution.
Last revised: 9 Oct 2024 / Arxiv.com
Retrieval-Augmented Decision Transformer: External Memory for In-context RL
In-context learning (ICL) is the ability of a model to learn a new task by observing a few exemplars in its context. While prevalent in NLP, this capability has recently also been observed in Reinforcement Learning (RL) settings
Last revised: 8 Oct 2024 / Arxiv.com
Parameter Choice and Neuro-Symbolic Approaches for Deep Domain-Invariant Learning
As artificial intelligence (AI) systems advance, we move towards broad AI: systems capable of performing well on diverse tasks, understanding context, and adapting rapidly to new scenarios. A central challenge for broad AI systems is to generalize over tasks in related domains and being robust to distribution shifts.
Last revised: 1 Oct 2024 / Arxiv.com
Large Language Models Can Self-Improve At Web Agent Tasks
Training models to act as agents that can effectively navigate and perform actions in a complex environment, such as a web browser, has typically been challenging due to lack of training data.
Last revised: 21 Aug 2024 / Arxiv.com
SymbolicAI: A framework for logic-based approaches combining generative models and solvers
We introduce SymbolicAI, a versatile and modular framework employing a logic-based approach to concept learning and flow management in generative processes.
Last revised: 2 May 2023 / Arxiv.com
Addressing Parameter Choice Issues in Unsupervised Domain Adaptation by Aggregation
We study the problem of choosing algorithm hyper-parameters in unsupervised domain adaptation, i.e., with labeled data in a source domain and unlabeled data in a target domain, drawn from a different input distribution.
Last revised: 9 Oct 2024 / Arxiv.com
Retrieval-Augmented Decision Transformer: External Memory for In-context RL
In-context learning (ICL) is the ability of a model to learn a new task by observing a few exemplars in its context. While prevalent in NLP, this capability has recently also been observed in Reinforcement Learning (RL) settings
Last revised: 8 Oct 2024 / Arxiv.com
Parameter Choice and Neuro-Symbolic Approaches for Deep Domain-Invariant Learning
As artificial intelligence (AI) systems advance, we move towards broad AI: systems capable of performing well on diverse tasks, understanding context, and adapting rapidly to new scenarios. A central challenge for broad AI systems is to generalize over tasks in related domains and being robust to distribution shifts.
Last revised: 1 Oct 2024 / Arxiv.com
Large Language Models Can Self-Improve At Web Agent Tasks
Training models to act as agents that can effectively navigate and perform actions in a complex environment, such as a web browser, has typically been challenging due to lack of training data.
Last revised: 21 Aug 2024 / Arxiv.com
SymbolicAI: A framework for logic-based approaches combining generative models and solvers
We introduce SymbolicAI, a versatile and modular framework employing a logic-based approach to concept learning and flow management in generative processes.
Last revised: 2 May 2023 / Arxiv.com
Addressing Parameter Choice Issues in Unsupervised Domain Adaptation by Aggregation
We study the problem of choosing algorithm hyper-parameters in unsupervised domain adaptation, i.e., with labeled data in a source domain and unlabeled data in a target domain, drawn from a different input distribution.
Last revised: 9 Oct 2024 / Arxiv.com
Retrieval-Augmented Decision Transformer: External Memory for In-context RL
In-context learning (ICL) is the ability of a model to learn a new task by observing a few exemplars in its context. While prevalent in NLP, this capability has recently also been observed in Reinforcement Learning (RL) settings
Last revised: 8 Oct 2024 / Arxiv.com
Parameter Choice and Neuro-Symbolic Approaches for Deep Domain-Invariant Learning
As artificial intelligence (AI) systems advance, we move towards broad AI: systems capable of performing well on diverse tasks, understanding context, and adapting rapidly to new scenarios. A central challenge for broad AI systems is to generalize over tasks in related domains and being robust to distribution shifts.
Last revised: 1 Oct 2024 / Arxiv.com
Large Language Models Can Self-Improve At Web Agent Tasks
Training models to act as agents that can effectively navigate and perform actions in a complex environment, such as a web browser, has typically been challenging due to lack of training data.
Last revised: 21 Aug 2024 / Arxiv.com
SymbolicAI: A framework for logic-based approaches combining generative models and solvers
We introduce SymbolicAI, a versatile and modular framework employing a logic-based approach to concept learning and flow management in generative processes.
Last revised: 2 May 2023 / Arxiv.com
Addressing Parameter Choice Issues in Unsupervised Domain Adaptation by Aggregation
We study the problem of choosing algorithm hyper-parameters in unsupervised domain adaptation, i.e., with labeled data in a source domain and unlabeled data in a target domain, drawn from a different input distribution.
Last revised: 9 Oct 2024 / Arxiv.com
Retrieval-Augmented Decision Transformer: External Memory for In-context RL
In-context learning (ICL) is the ability of a model to learn a new task by observing a few exemplars in its context. While prevalent in NLP, this capability has recently also been observed in Reinforcement Learning (RL) settings
Last revised: 8 Oct 2024 / Arxiv.com
Parameter Choice and Neuro-Symbolic Approaches for Deep Domain-Invariant Learning
As artificial intelligence (AI) systems advance, we move towards broad AI: systems capable of performing well on diverse tasks, understanding context, and adapting rapidly to new scenarios. A central challenge for broad AI systems is to generalize over tasks in related domains and being robust to distribution shifts.
Last revised: 1 Oct 2024 / Arxiv.com
Large Language Models Can Self-Improve At Web Agent Tasks
Training models to act as agents that can effectively navigate and perform actions in a complex environment, such as a web browser, has typically been challenging due to lack of training data.
Last revised: 21 Aug 2024 / Arxiv.com
SymbolicAI: A framework for logic-based approaches combining generative models and solvers
We introduce SymbolicAI, a versatile and modular framework employing a logic-based approach to concept learning and flow management in generative processes.
Last revised: 2 May 2023 / Arxiv.com
Addressing Parameter Choice Issues in Unsupervised Domain Adaptation by Aggregation
We study the problem of choosing algorithm hyper-parameters in unsupervised domain adaptation, i.e., with labeled data in a source domain and unlabeled data in a target domain, drawn from a different input distribution.
Last revised: 9 Oct 2024 / Arxiv.com
Retrieval-Augmented Decision Transformer: External Memory for In-context RL
In-context learning (ICL) is the ability of a model to learn a new task by observing a few exemplars in its context. While prevalent in NLP, this capability has recently also been observed in Reinforcement Learning (RL) settings
Last revised: 8 Oct 2024 / Arxiv.com
Parameter Choice and Neuro-Symbolic Approaches for Deep Domain-Invariant Learning
As artificial intelligence (AI) systems advance, we move towards broad AI: systems capable of performing well on diverse tasks, understanding context, and adapting rapidly to new scenarios. A central challenge for broad AI systems is to generalize over tasks in related domains and being robust to distribution shifts.
Last revised: 1 Oct 2024 / Arxiv.com
Large Language Models Can Self-Improve At Web Agent Tasks
Training models to act as agents that can effectively navigate and perform actions in a complex environment, such as a web browser, has typically been challenging due to lack of training data.
Last revised: 21 Aug 2024 / Arxiv.com
SymbolicAI: A framework for logic-based approaches combining generative models and solvers
We introduce SymbolicAI, a versatile and modular framework employing a logic-based approach to concept learning and flow management in generative processes.
Last revised: 2 May 2023 / Arxiv.com
Addressing Parameter Choice Issues in Unsupervised Domain Adaptation by Aggregation
We study the problem of choosing algorithm hyper-parameters in unsupervised domain adaptation, i.e., with labeled data in a source domain and unlabeled data in a target domain, drawn from a different input distribution.
Last revised: 9 Oct 2024 / Arxiv.com
Retrieval-Augmented Decision Transformer: External Memory for In-context RL
In-context learning (ICL) is the ability of a model to learn a new task by observing a few exemplars in its context. While prevalent in NLP, this capability has recently also been observed in Reinforcement Learning (RL) settings
Last revised: 8 Oct 2024 / Arxiv.com
Parameter Choice and Neuro-Symbolic Approaches for Deep Domain-Invariant Learning
As artificial intelligence (AI) systems advance, we move towards broad AI: systems capable of performing well on diverse tasks, understanding context, and adapting rapidly to new scenarios. A central challenge for broad AI systems is to generalize over tasks in related domains and being robust to distribution shifts.
Last revised: 1 Oct 2024 / Arxiv.com
Large Language Models Can Self-Improve At Web Agent Tasks
Training models to act as agents that can effectively navigate and perform actions in a complex environment, such as a web browser, has typically been challenging due to lack of training data.
Last revised: 21 Aug 2024 / Arxiv.com
SymbolicAI: A framework for logic-based approaches combining generative models and solvers
We introduce SymbolicAI, a versatile and modular framework employing a logic-based approach to concept learning and flow management in generative processes.
Last revised: 2 May 2023 / Arxiv.com
Addressing Parameter Choice Issues in Unsupervised Domain Adaptation by Aggregation
We study the problem of choosing algorithm hyper-parameters in unsupervised domain adaptation, i.e., with labeled data in a source domain and unlabeled data in a target domain, drawn from a different input distribution.
Last revised: 9 Oct 2024 / Arxiv.com
Retrieval-Augmented Decision Transformer: External Memory for In-context RL
In-context learning (ICL) is the ability of a model to learn a new task by observing a few exemplars in its context. While prevalent in NLP, this capability has recently also been observed in Reinforcement Learning (RL) settings
Last revised: 8 Oct 2024 / Arxiv.com
Parameter Choice and Neuro-Symbolic Approaches for Deep Domain-Invariant Learning
As artificial intelligence (AI) systems advance, we move towards broad AI: systems capable of performing well on diverse tasks, understanding context, and adapting rapidly to new scenarios. A central challenge for broad AI systems is to generalize over tasks in related domains and being robust to distribution shifts.
Last revised: 1 Oct 2024 / Arxiv.com
Large Language Models Can Self-Improve At Web Agent Tasks
Training models to act as agents that can effectively navigate and perform actions in a complex environment, such as a web browser, has typically been challenging due to lack of training data.
Last revised: 21 Aug 2024 / Arxiv.com
SymbolicAI: A framework for logic-based approaches combining generative models and solvers
We introduce SymbolicAI, a versatile and modular framework employing a logic-based approach to concept learning and flow management in generative processes.
Last revised: 2 May 2023 / Arxiv.com
Addressing Parameter Choice Issues in Unsupervised Domain Adaptation by Aggregation
We study the problem of choosing algorithm hyper-parameters in unsupervised domain adaptation, i.e., with labeled data in a source domain and unlabeled data in a target domain, drawn from a different input distribution.
Last revised: 9 Oct 2024 / Arxiv.com
Retrieval-Augmented Decision Transformer: External Memory for In-context RL
In-context learning (ICL) is the ability of a model to learn a new task by observing a few exemplars in its context. While prevalent in NLP, this capability has recently also been observed in Reinforcement Learning (RL) settings
Last revised: 8 Oct 2024 / Arxiv.com
Parameter Choice and Neuro-Symbolic Approaches for Deep Domain-Invariant Learning
As artificial intelligence (AI) systems advance, we move towards broad AI: systems capable of performing well on diverse tasks, understanding context, and adapting rapidly to new scenarios. A central challenge for broad AI systems is to generalize over tasks in related domains and being robust to distribution shifts.
Last revised: 1 Oct 2024 / Arxiv.com
Large Language Models Can Self-Improve At Web Agent Tasks
Training models to act as agents that can effectively navigate and perform actions in a complex environment, such as a web browser, has typically been challenging due to lack of training data.
Last revised: 21 Aug 2024 / Arxiv.com
SymbolicAI: A framework for logic-based approaches combining generative models and solvers
We introduce SymbolicAI, a versatile and modular framework employing a logic-based approach to concept learning and flow management in generative processes.
Last revised: 2 May 2023 / Arxiv.com
Addressing Parameter Choice Issues in Unsupervised Domain Adaptation by Aggregation
We study the problem of choosing algorithm hyper-parameters in unsupervised domain adaptation, i.e., with labeled data in a source domain and unlabeled data in a target domain, drawn from a different input distribution.
Last revised: 9 Oct 2024 / Arxiv.com
Retrieval-Augmented Decision Transformer: External Memory for In-context RL
In-context learning (ICL) is the ability of a model to learn a new task by observing a few exemplars in its context. While prevalent in NLP, this capability has recently also been observed in Reinforcement Learning (RL) settings
Last revised: 8 Oct 2024 / Arxiv.com
Parameter Choice and Neuro-Symbolic Approaches for Deep Domain-Invariant Learning
As artificial intelligence (AI) systems advance, we move towards broad AI: systems capable of performing well on diverse tasks, understanding context, and adapting rapidly to new scenarios. A central challenge for broad AI systems is to generalize over tasks in related domains and being robust to distribution shifts.
Last revised: 1 Oct 2024 / Arxiv.com
Large Language Models Can Self-Improve At Web Agent Tasks
Training models to act as agents that can effectively navigate and perform actions in a complex environment, such as a web browser, has typically been challenging due to lack of training data.
Last revised: 21 Aug 2024 / Arxiv.com
SymbolicAI: A framework for logic-based approaches combining generative models and solvers
We introduce SymbolicAI, a versatile and modular framework employing a logic-based approach to concept learning and flow management in generative processes.
Last revised: 2 May 2023 / Arxiv.com
Addressing Parameter Choice Issues in Unsupervised Domain Adaptation by Aggregation
We study the problem of choosing algorithm hyper-parameters in unsupervised domain adaptation, i.e., with labeled data in a source domain and unlabeled data in a target domain, drawn from a different input distribution.
Last revised: 9 Oct 2024 / Arxiv.com
Retrieval-Augmented Decision Transformer: External Memory for In-context RL
In-context learning (ICL) is the ability of a model to learn a new task by observing a few exemplars in its context. While prevalent in NLP, this capability has recently also been observed in Reinforcement Learning (RL) settings
Last revised: 8 Oct 2024 / Arxiv.com
Parameter Choice and Neuro-Symbolic Approaches for Deep Domain-Invariant Learning
As artificial intelligence (AI) systems advance, we move towards broad AI: systems capable of performing well on diverse tasks, understanding context, and adapting rapidly to new scenarios. A central challenge for broad AI systems is to generalize over tasks in related domains and being robust to distribution shifts.
Last revised: 1 Oct 2024 / Arxiv.com
Large Language Models Can Self-Improve At Web Agent Tasks
Training models to act as agents that can effectively navigate and perform actions in a complex environment, such as a web browser, has typically been challenging due to lack of training data.
Last revised: 21 Aug 2024 / Arxiv.com
SymbolicAI: A framework for logic-based approaches combining generative models and solvers
We introduce SymbolicAI, a versatile and modular framework employing a logic-based approach to concept learning and flow management in generative processes.
Last revised: 2 May 2023 / Arxiv.com
Addressing Parameter Choice Issues in Unsupervised Domain Adaptation by Aggregation
We study the problem of choosing algorithm hyper-parameters in unsupervised domain adaptation, i.e., with labeled data in a source domain and unlabeled data in a target domain, drawn from a different input distribution.
Last revised: 9 Oct 2024 / Arxiv.com
Retrieval-Augmented Decision Transformer: External Memory for In-context RL
In-context learning (ICL) is the ability of a model to learn a new task by observing a few exemplars in its context. While prevalent in NLP, this capability has recently also been observed in Reinforcement Learning (RL) settings
Last revised: 8 Oct 2024 / Arxiv.com
Parameter Choice and Neuro-Symbolic Approaches for Deep Domain-Invariant Learning
As artificial intelligence (AI) systems advance, we move towards broad AI: systems capable of performing well on diverse tasks, understanding context, and adapting rapidly to new scenarios. A central challenge for broad AI systems is to generalize over tasks in related domains and being robust to distribution shifts.
Last revised: 1 Oct 2024 / Arxiv.com
Large Language Models Can Self-Improve At Web Agent Tasks
Training models to act as agents that can effectively navigate and perform actions in a complex environment, such as a web browser, has typically been challenging due to lack of training data.
Last revised: 21 Aug 2024 / Arxiv.com
SymbolicAI: A framework for logic-based approaches combining generative models and solvers
We introduce SymbolicAI, a versatile and modular framework employing a logic-based approach to concept learning and flow management in generative processes.
Last revised: 2 May 2023 / Arxiv.com
Addressing Parameter Choice Issues in Unsupervised Domain Adaptation by Aggregation
We study the problem of choosing algorithm hyper-parameters in unsupervised domain adaptation, i.e., with labeled data in a source domain and unlabeled data in a target domain, drawn from a different input distribution.
Last revised: 9 Oct 2024 / Arxiv.com
Retrieval-Augmented Decision Transformer: External Memory for In-context RL
In-context learning (ICL) is the ability of a model to learn a new task by observing a few exemplars in its context. While prevalent in NLP, this capability has recently also been observed in Reinforcement Learning (RL) settings
Last revised: 8 Oct 2024 / Arxiv.com
Parameter Choice and Neuro-Symbolic Approaches for Deep Domain-Invariant Learning
As artificial intelligence (AI) systems advance, we move towards broad AI: systems capable of performing well on diverse tasks, understanding context, and adapting rapidly to new scenarios. A central challenge for broad AI systems is to generalize over tasks in related domains and being robust to distribution shifts.
Last revised: 1 Oct 2024 / Arxiv.com
Large Language Models Can Self-Improve At Web Agent Tasks
Training models to act as agents that can effectively navigate and perform actions in a complex environment, such as a web browser, has typically been challenging due to lack of training data.
Get news and product updates
ExtensityAI FlexCo
Wels, Austria
office@extensity.ai
INDUSTRIES
CUSTOMER STORIES
Get news and product updates
ExtensityAI FlexCo
Wels, Austria
office@extensity.ai
INDUSTRIES
CUSTOMER STORIES
Get news and product updates
ExtensityAI FlexCo
Wels, Austria
office@extensity.ai
INDUSTRIES
CUSTOMER STORIES
Industries
Industries